Decoding Protein Interactions with Domain-Aware Machine Learning
Identifying protein interactions can help discover new medicines
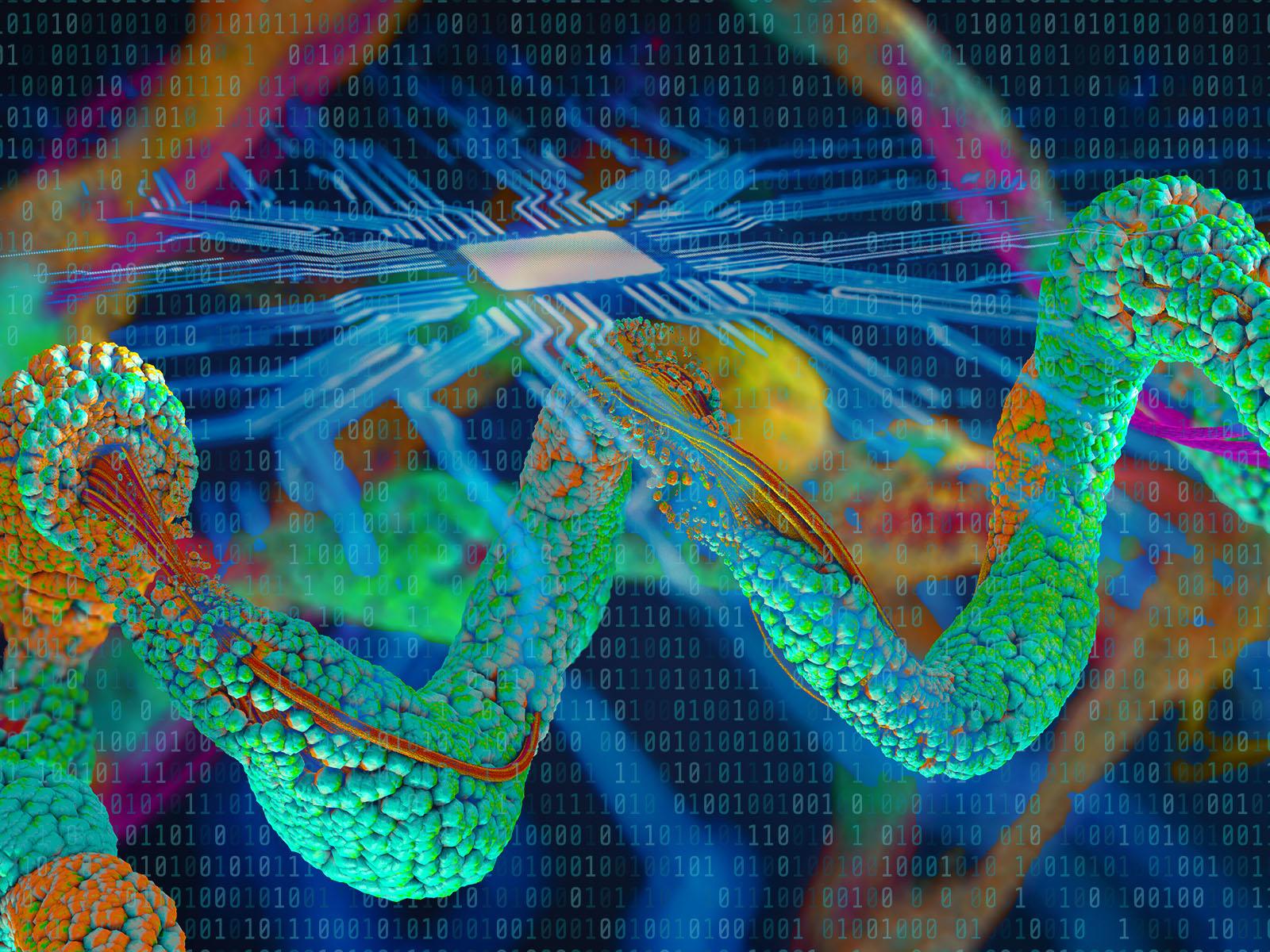
A new domain-aware machine learning technique can identify protein-ligand interactions—a critical step in the drug discovery process.
(Illustration by Cortland Johnson | Pacific Northwest National Laboratory)
The drug design process can take many years. Predicting protein interactions with other biomolecules is an increasingly critical step in this process. Researchers at Pacific Northwest National Laboratory (PNNL) leveraged domain-aware machine learning to make it faster. A team consisting of Carter Knutson, Mridula Bontha, Jenna (Bilbrey) Pope, and led by Neeraj Kumar, created two distinct graph neural networks (GNNs) capable of identifying protein-ligand interactions that lead to higher binding affinity and bioactivity. Their research was published in Nature’s Scientific Reports.
These GNNs consider experimentally resolved 3-D atomic structures of both the candidate drug molecule and the potential protein target to learn about the protein’s interactions with small molecules. From this information, the models can predict the binding affinity and the biophysical properties (such as IC50) of small molecules to their protein targets—a crucial component that helps to determine the efficacy of a drug.
“Experimentally measuring binding affinities and biophysical properties is one of the greatest bottlenecks in drug discovery,” said Kumar, data scientist and program development lead in the Biological Sciences Division. “We curated 3-D protein structural data and devised GNNs as a means to predict protein-ligand interactions with high accuracy correlated with experimental properties to aid in this process.”

These models are especially useful in the early stages of protein functional discovery, and drug development, where the GNNs can screen large numbers of candidate molecules for their potency against a specific target. “With the invention of AlphaFold2 from DeepMind, the GNN model can take their predicted 3-D protein structures as an input to identify therapeutic candidates for new and emerging pathogens in order to expedite the drug design process,” said Kumar.
Since the beginning of the COVID-19 pandemic, PNNL researchers used computational means to expedite drug discovery against SARS-CoV-2. Kumar and the rest of the team built on this multidisciplinary research by testing their models against two SARS-CoV-2 proteins—which Kumar was already familiar with through his work with the Department of Energy’s National Virtual Biotechnology Laboratory.
Thanks to the Mathematics for Artificial Reasoning in Science (MARS) initiative and Laboratory Directed Research and Development programs at PNNL, Kumar was able to build a cross disciplinary team including Dr. Pope, data scientist from PNNL’s Computing and Analytics Division, to expand this work.
“The MARS initiative has been great for fostering collaborations to get together diverse groups of researchers to develop domain aware and explainable AI systems capable of tackling difficult scientific problems such as protein interaction prediction,” said Pope. “I’m excited to see where such atom-scale GNNs will lead us in the future.”
Published: May 16, 2022