ICLR 2024
Pacific Northwest National Laboratory to present insights at the Twelfth International Conference on Learning Representations
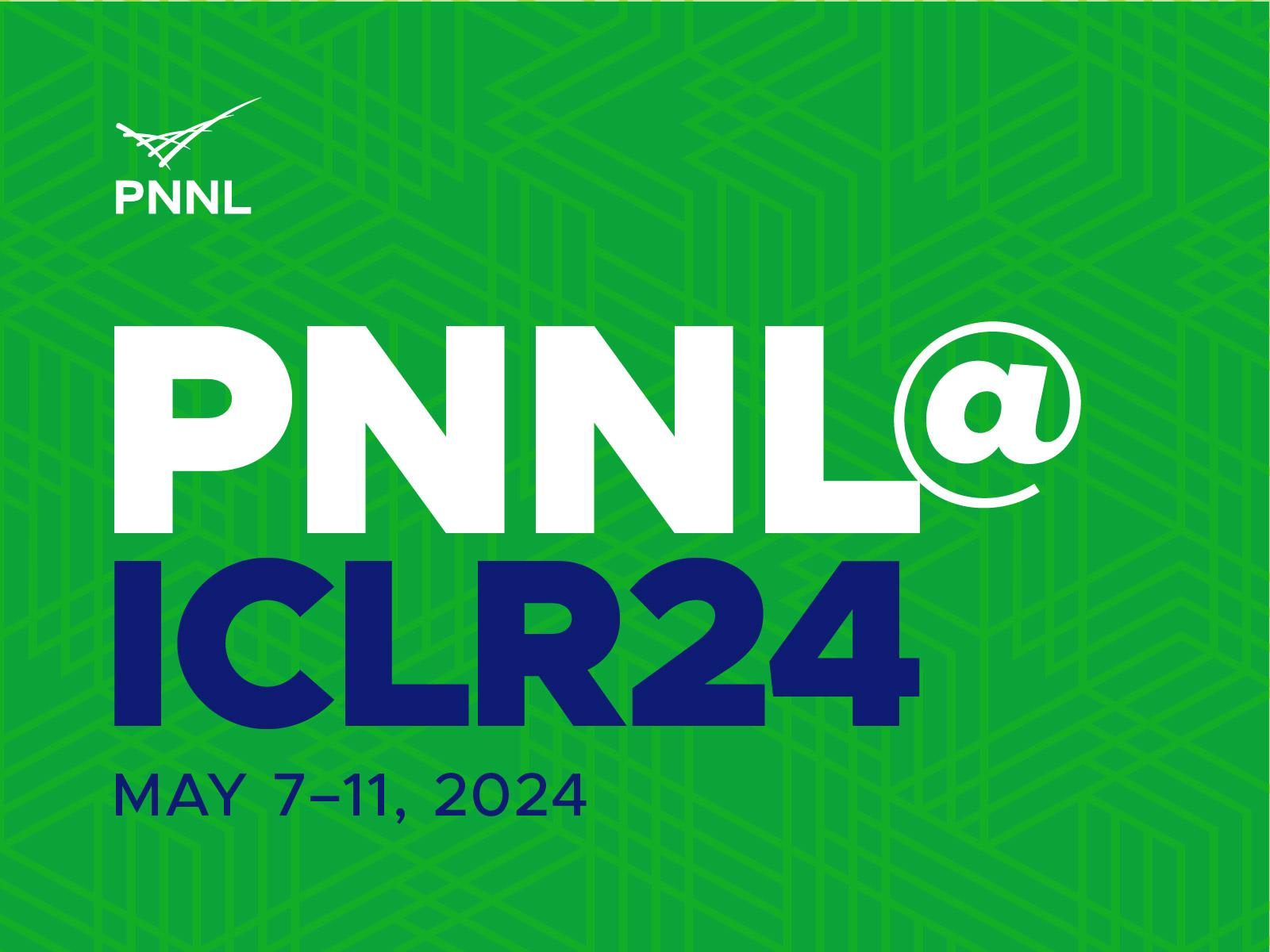
May 7 – 11, 2024
Vienna, Austria
Lab-Level Communications Priority Topics
Computing
Pacific Northwest National Laboratory to present insights at the Twelfth International Conference on Learning Representations
Vienna, Austria
Pacific Northwest National Laboratory (PNNL) will be at the Twelfth International Conference on Learning Representations (ICLR), May 7-11, 2024, in Vienna, Austria. ICLR brings together researchers dedicated to advancing representation learning, a branch of artificial intelligence (AI).
The remarkable advancements in machine learning (ML) and artificial intelligence (AI) have paved the way for a new era of applications, revolutionizing various aspects of our daily lives. From situational awareness to analyzing and detecting threats and interpreting online signals to ensure system reliability, PNNL researchers are at the forefront of scientific exploration and national security, harnessing the power of AI to tackle complex scientific problems.
PNNL authors: Andrew Engel, Tony Chiang, and Sutanay Choudhury
Summary: One of the recent advances to explainable AI has come through surrogate modeling, where neural networks (NNs) are approximated as simpler ML algorithms, such as kernel-machines. Meanwhile, explain-by-example algorithms have employed various kernel functions to investigate a diverse set of NN behavior, including attribution of classification decisions to training data. In this work, we combine these two advances to analyze approximate neural tangent kernel (NTK) for data attribution. We search kernel functions to find a faithful surrogate model of the underlying NN and demonstrate the efficacy of surrogate linear feature spaces to NNs through a rank-based correlation measure of the surrogate model’s ability to replicate the NN decision confidences. We investigate randomly projected variants of approximate NTK that can be computed in about 10 minutes for a ResNet18 model on CIFAR10. Based on these observations, we conclude that kernel linear models are effective surrogates of NNs and that the introduced trace-NTK kernels are the best performing of all studied.
PNNL author: Ang Li
Summary: Nature performs complex computations constantly at clearly lower cost and higher performance than digital computers. It is crucial to understand how to harness the unique computational power of nature in ML. In the past decade, besides the development of NNs, the community has also relentlessly explored nature-powered ML paradigms. Although most of them are still predominantly theoretical, a new practical paradigm enabled by the recent advent of CMOS-compatible room-temperature, nature-based computers has emerged. By harnessing the nature's power of entropy increase, this paradigm can solve binary learning problems delivering immense speedup and energy savings compared with NNs, while maintaining comparable accuracy. Regrettably, its values to the real world are highly constrained by its binary nature. A clear pathway to its extension to real-valued problems remains elusive. This paper aims to unleash this pathway by proposing a novel end-to-end nature-powered graph learning (NP-GL) framework. Specifically, through a three-dimensional co-design, NP-GL can leverage the nature's power of entropy increase to efficiently solve real-valued graph learning problems. Experimental results across four real-world applications with six datasets demonstrate that NP-GL delivers, on average, 6970X speedup and 10^5x energy consumption reduction with comparable or even higher accuracy than graph NNs.
PNNL authors: Amanda Howard, Saad Qadeer, Andrew Engel, Adam Tsou, Max Vargas, Tony Chiang, Panos Stinis
Summary: Recent work has shown that the empirical NTK can significantly improve the training of physics-informed deep operator network (DeepONets). The NTK, however, is costly to calculate, greatly increasing the cost to train such systems. In this paper, we study the performance of the empirical conjugate kernel (CK) for physics-informed DeepONets, an efficient approximation to the NTK that has been observed to yield similar results. For physics-informed DeepONets, we show that the CK performance is comparable to the NTK, while significantly reducing time complexity for training DeepONets.
If you’re looking for a career at PNNL, check out our current openings: